Interviews
Carl Rost, Principal Consultant at Patsnap – Interview Series
Carl Rost is the mind behind the AI-powered patent search tools at Patsnap.
Patsnap stands at the forefront of innovation intelligence, harnessing the power of AI and machine learning to sift through billions of datasets, enabling innovators to make crucial connections. Their cutting-edge LLM technology, tailored for R&D and IP professionals, effortlessly navigates through billions of pages of patents daily. Patsnap’s AI assistant engages in conversational responses to novelty questions and can pinpoint specific answers within extensive texts. For instance, it can accurately determine whether a particular widget type is already patented.
Can you provide an overview of how Patsnap's AI assistant works and its primary functions?
Sure! It’s an AI assistant called Hiro that allows you to ask questions about a specific patent or even a result set or our entire database! It’s been trained to understand innovation and patent related questions and respond in a way that satisfies technical subject matter experts and IP professionals. A recent advancement is that Hiro can even help you solve technical problems and propose novel directions for new inventions by applying inventive principles to technical solutions and problems that have been found in our patent and literature database. Hiro works a bit differently depending if you use it in our products that are for R&D or for IP professionals.
I think what makes Hiro unique is that it’s powered by Patsnap’s proprietary LLM, answers also link references and sources from Patsnap’s library of 200 million patents, 190 million pieces of literature, 254 million chemical structures, 879 million biological sequences, and 2 billion news articles.
What problems is this application solving for enterprises?
Great innovators should spend their time innovating, not determining novelty of products or doing preliminary research of the market. Patent data is one of our richest sources of technical information, rivaling journal data, especially in certain technology fields. For R&D, the time it takes to find and interrogate this type of data has been a massive blocker to leverage this, but tools like Hiro can truly democratize this type of information for the first time.
For legal professionals, it's common to spend hours, days, weeks, running prior art and freedom to operate searches. With AI tools this can be done more quickly, and with more accuracy, freeing up bandwidth for more strategic work.
Existing AI tools are one of two things: overly generalized and therefore not appropriate for the intellectual property space, or they are black boxes, with no transparency as to resources, reducing confidence and obstructing decision-making. With Hiro, we link back to sources and ensure full visibility at all stages of the development process.
What were the main challenges your team faced while developing the AI features for Patsnap, and how did you overcome them?
We know that individuals building new inventions want to keep them protected, so security was top of mind when building Hiro. As the model powering Hiro is local and built into our app, no data leaves the environment to third parties that are hard to trust. Our competitors didn’t do the groundwork and bolted on third party models that don’t stand up to scrutiny. When we say that we aren’t training models on customer data, we know that to be true and can show our customers that and what we do instead. In contrast, our competitors' solutions expose you to risk through third parties who have a less than stellar reputation, in terms of transparency and handling of data.
Could you elaborate on how Hiro answers specific novelty questions and the impact this has on R&D and IP workflows?
With Hiro, users can ask questions like “What aspects of this invention make it novel?” or “How might this patent hold up in different legal systems?” or even “how to build a wearable jetpack” and get answers that speak to each step of the invention process. Compared to generalist models, Hiro really gets what makes a patent special. Users don’t need to be patent experts to get to the bottom of what is or isn’t novel within their invention, and can understand in seconds which part of their product or tool needs to be protected.
How does Hiro handle the vast amount of data from patents and non-patent literature to provide precise and relevant answers?
We did extensive training on that dataset, and rated the responses with experts. We then trained AI on the expert responses, had the AI rate output, and had experts review that. All in all, we’ve rated millions of data points this way to ensure the responses are meaningful for tech experts and patent pros.
How does Hiro utilize large language models (LLMs) to enhance the efficiency of patent searches and IP analysis? What types of data were used to train Patsnap's proprietary LLM, and how do you ensure its accuracy and reliability?
Patsnap built an industry-specific LLM to power Hiro. The LLM has been trained on patent records, academic papers, and other innovation data, which helps it understand and retell info in a way that is more helpful to professionals than generalist models. To ensure accuracy and reliability, we employed rigorous data preprocessing methods, including filtering out lowquality data, deduplication, and rewriting. We also synthesized new data by combining different sources to enhance the model's understanding of IP-specific nuances. We supervised finetuning and reinforcement learning from human feedback to continually improve its performance.
PatsnapGPT has been tested extensively and has outperformed GPT-4 in IP-specific tasks, demonstrating superior capabilities in drafting, classifying, summarizing, and reasoning within the patent domain.
The proprietary LLM is transparent, linking sources and references, and it’s not trained on customer data. It’s the only industry player using an in-house tuned LLM, in an industry that is especially reliant on data privacy and confidentiality.
How does Patsnap’s proprietary LLM compare to other general-purpose LLMs like GPT-4 in terms of performance and accuracy for IP-related tasks?
Patsnap’s proprietary LLM outperforms GPT-4 when it comes to intellectual property queries. Using the USPTO Patent Bar Exam, PatsnapGPT-1.0’s performed at the level of an IP expert, while general LLMs did not reach the cutoff for patent lawyers taking the exam.
PatsnapGPT really stands out when you look at how it performs in IP-specific benchmarks. Hiro consistently scores higher than general models like GPT-4 on the USPTO Patent Bar Exam. General LLMs fail to pass the 70-point cutoff on the exam, while PatsnapGPT 1.0 scored at the level of an IP expert. This shows it has a better grasp of IP fundamentals. Additionally, in the PatentBench, which is a comprehensive benchmark for IP tasks, PatsnapGPT excelled in several areas. It produced more accurate and relevant texts for patent writing, scored higher in classifying patents according to the International Patent Classification system, and its summaries of technical effects, problems, methods, and abstracts were consistently rated higher by evaluators. It also shows faster speeds and lower memory usage compared to GPT-4 for long patent documents.
How do you envision the role of AI evolving in the field of intellectual property and research and development over the next decade?
I see AI playing an increasingly central role in intellectual property and research and development over the next decade. For one, AI will greatly enhance the efficiency and accuracy of patent searches and analysis. Advanced AI models like PatsnapGPT will become even better at understanding and categorizing complex technical documents, drafting high quality patent specifications, and identifying potential infringements or overlaps in existing patents. This will save a tremendous amount of time and reduce the margin for human error.
Moreover, AI will revolutionize how we handle and interpret vast amounts of IP data. With the ability to process and analyze large datasets quickly, AI can uncover trends and insights that might otherwise go unnoticed. This can inform better decision-making and strategy in IP management and R&D, such as identifying emerging technologies, potential areas for innovation, and strategic partnerships.
In R&D, AI will drive innovation by aiding in the discovery process. Machine learning algorithms can analyze previous research, predict outcomes, and even suggest new lines of inquiry, accelerating the pace of discovery and development. AI can also simulate experiments and model complex systems, reducing the need for costly and time-consuming physical trials.
As AI technology continues to evolve, its integration into IP and R&D will enhance creativity, efficiency, and strategic planning.
Thank you for the great interview, readers who wish to learn more should visit Patsnap.
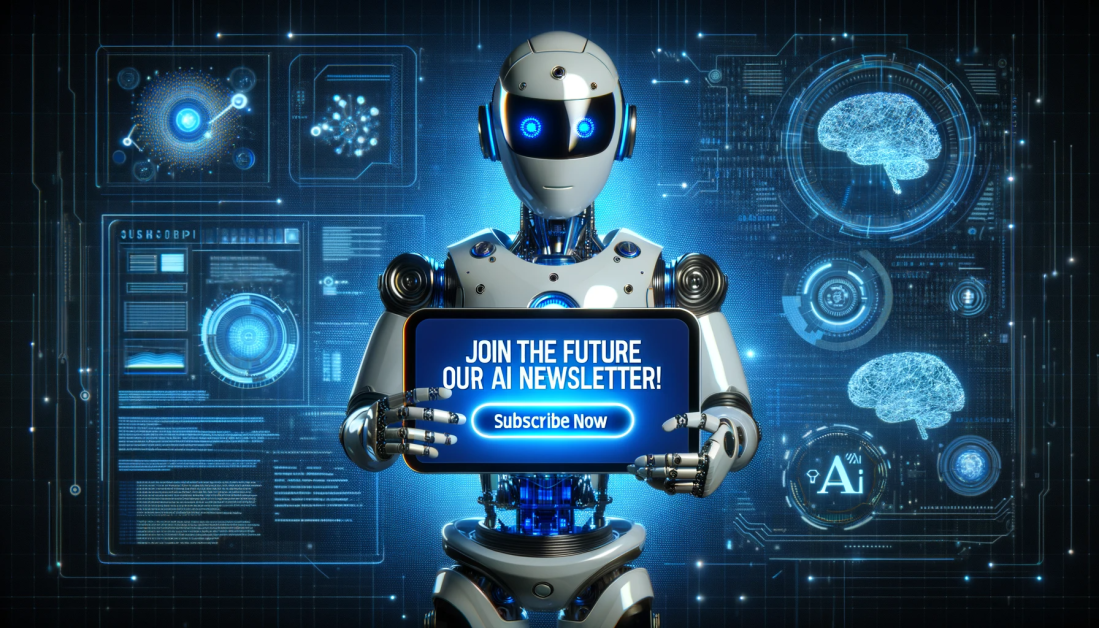
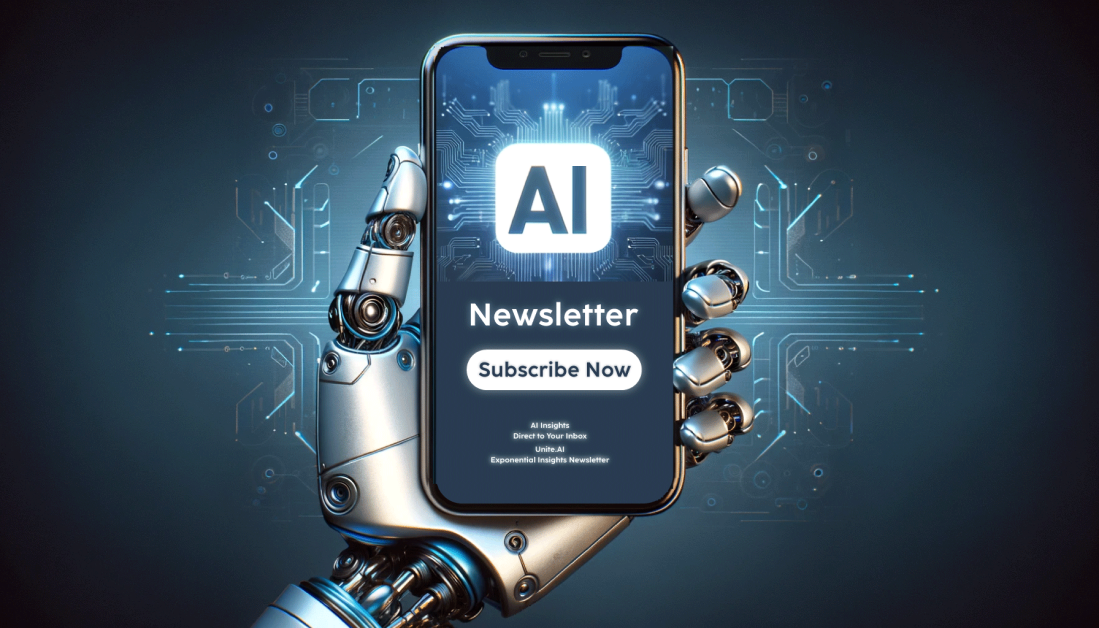